Meta has recently released an AI model called SeamlessM4T which can transcribe and translate close to 100 languages across both text and speech.
The new AI model is available in open source along with SeamlessAlign, a new translation dataset. Meta stated that SeamlessM4T represents a “significant breakthrough” in the field of AI-powered, speech-to-text, and speech-to-speech. Meta claims that the new system is the first all-in-one multilingual multimodal AI translation and transcription model.
“Our single model provides on-demand translations that enable people who speak different languages to communicate more effectively,” Meta writes in a blog post. “SeamlessM4T implicitly recognizes the source languages without the need for a separate language identification model.”
SeamlessM4T is a successor to Meta’s No Language Left Behind, a text-to-text machine translation model that supports 200 languages. It also builds on Massively Multilingual Speech, Meta’s framework that provides speech recognition, speech synthesis tech, and language identification across more than 1,100 languages.
While developing the AI model, Meta scraped publicly available text (in the order of “tens of billions” of sentences) and 4 million hours of speech from the web. Though the company hasn’t cited specifically where they collected the data to train the model, they stated it wasn’t copyrighted and came from open-source or licensed sources.
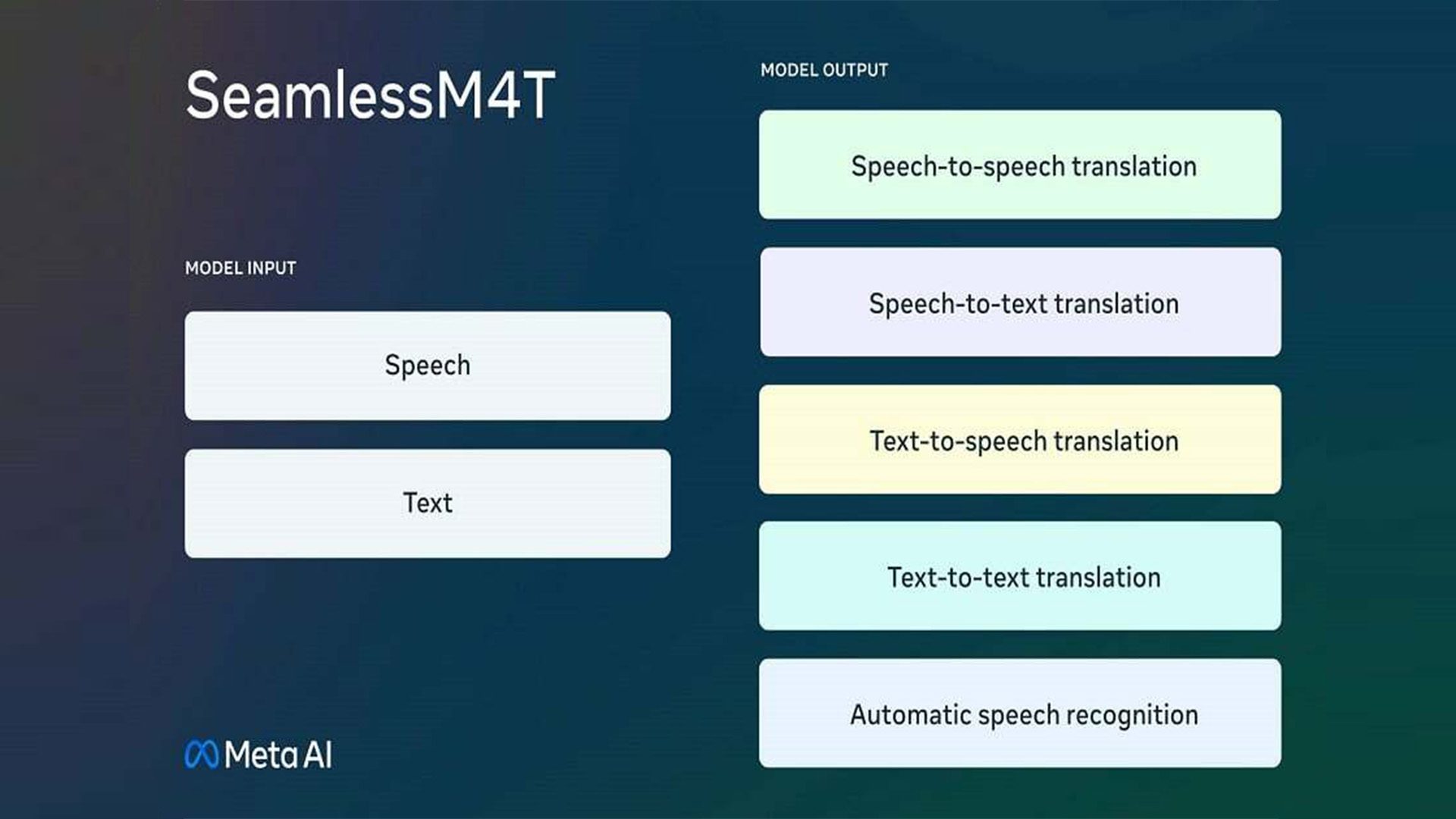
This new AI model comes at a time when many big companies are attempting to develop language translation and transcription models, including Amazon, Microsoft, and OpenAI. Google is also creating a Universal Speech Model which is part of a larger effort to build a model that can the world’s 1,000 most spoken languages. Additionally, Mozilla spearheaded the project titled Common Voice, one of the largest multi-language collections of voices that can be used to train automatic speech recognition algorithms
The training dataset for the SeamlessM4T, dubbed SeamlessAlign, involved researchers aligning 443,000 hours of speech with texts and creating 29,000 hours of “speech-to-speech” alignments. This taught the model to translate text, transcribe speech to text, generate speech from text, and translate words spoken in one language to words in another language.
Meta also claims that, on an internal benchmark, the SeamlessM4T was able to perform better against “speaker variations” and background noises in speech-to-text tasks compared to the current state-of-the-art transcription models.
“With state-of-the-art results, we believe SeamlessM4T is an important breakthrough in the AI community’s quest toward creating universal multitask systems,” Meta wrote in the blog post.
As with other AI translation systems, there are some forms of bias that make the system imperfect. For example, in a whitepaper published alongside the blog post, Meta reveals that the model “overgeneralizes to masculine forms when translating from neutral terms” and performs better when translating from the masculine reference (e.g. nouns like “he” in English) for most languages. Additionally, SeamlessM4T reportedly prefers translating the masculine form about 10% of the time — perhaps due to an “overrepresentation of masculine lexica” in the training data.
For this reason, Meta advises against using SeamlessM4T for long-form translation and certified translations such as those recognized by translation authorities and government agencies.
“This single system approach reduces errors and delays, increasing the efficiency and quality of the translation process, bringing us closer to making seamless translation possible,” stated Juan Pino, a research scientist at Meta’s AI research division and a contributor to the project. “In the future, we want to explore how this foundational model can enable new communication capabilities — ultimately bringing us closer to a world where everyone can be understood.”